lastmod: 11 May, 2020
Please, find the presentation for the students’ seminary here
Notice: the link above might not be working until the actual presentation date or, if working, the presentation may suffer modifications until the seminary’s date.
This is not the presentation for the Department meeting.
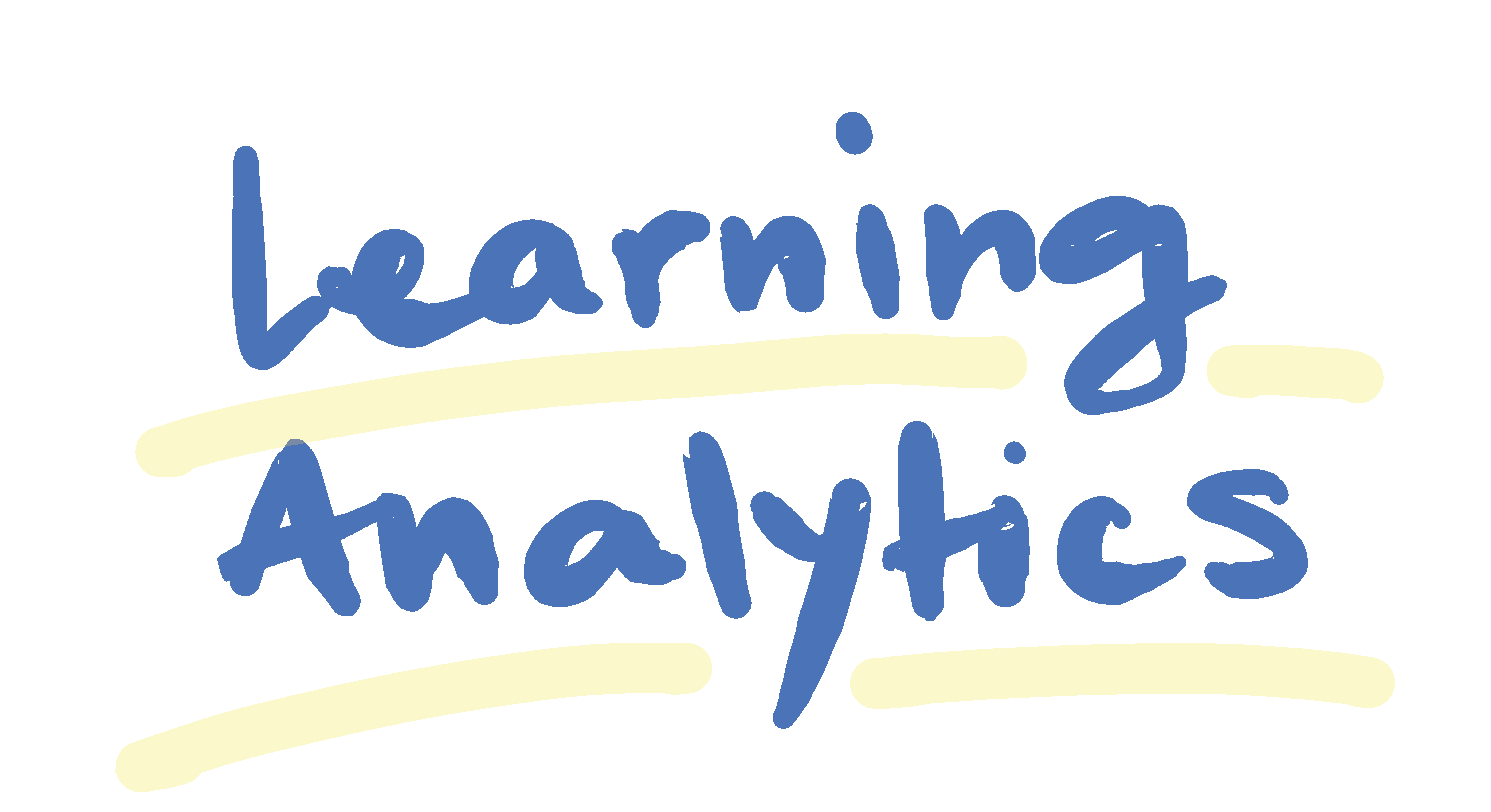
Learning Analytics involves “measurement, collection, analysis, and reporting of data ahout learners and their contexts for purposes of understanding learners and optimizing learning environments”.
(Fischer, Hmelo-Silver, Goldman, & Reimann, 2018; Siemens & Baker, 2012)
Academic Analytics is defined as “the intersection of technology, information, management culture and the application of information to manage the academic enterprise”.
(Goldstein & Katz, 2005)
The words of Turing award winner, Jim Gray, “everything about science is changing because of the impact of information technology”, echo strongly these days in the scientific world. There is virtually no scientific field or praxeology left today that can afford to ignore the availability of computational technology and of Big Data.
The main aims of science have always been to make (accurate) predictions and explain why they happen. But with the current mindboggling precision of machine learning and ability to work ‘in the blind’, i.e., agnostic to theories and assumptions, some might say that ‘classical statistics’ only took us this far, while other may even put a shade of post-truth on it, and take the pragmatical approach, “I don’t care about the ‘why’, insofar as I know the ‘what’”.
Learning Sciences had no shelter in the face of the Data Science tsunami, and it is no surprise why. While not substituting epistemology in this regard, it come close in the way it endures tremendous pressure, as a scientific field, to understand and validate “knowledge building”. Moreover, it produces both “brains”, for the society, and theories, for science. And, Learning Sciences do not have it easy. It must overcome several important challenges from the very onset, chief amongst them being that educational systems are giants with huge inertia and are inherently rigid because they are based on systems of beliefs.
First, as opposed to businesses, which are goal oriented, and not only free, but rather mandated to change their strategies as the market requires it, education systems are prisoners of their own culture production. They teach the way we do science; and some may see the new data-driven approaches as ‘giving in’, if not even as ‘pagan’ attempts to pervert true science. Secondly, staying competitive in Data Science, is not simply a matter of drawing borders in the sand and claiming the Learning Analytics hills, but a matter of vision and resources. Some fields in education, like the IT specialties and certain subfields of Business, are inherently riding the wave because of their built-in mechanism of study and practice. However, there are many others that risk missing the opportunities that Data Science bring into the game.
And this is what our presentation tries to look into, by raising several questions. How ready are Learning Sciences to take on Data Science? How can we reconcile our ‘classical’ way of building understanding of the world with the ‘black boxes” of machine learning? Are existing methods of research exclusive or complementary with respect to the new computational-based methods? What are the main trends today in the newly born Learning Analytics field? Is it all about Big Data and predicting trends or can it be used viably as a new approach to scientific research? Can we apply Learning Analytics to all subject matters and to all areas of scientific inquires or only to some? Who are the main pioneers in the field and how their vision sets the agenda?
While I dare argue that we are rather far from drawing conclusions, there are several trends that appear to set the agenda. First, the digital instrumentation of learning, or, more specifically, the technological scaffolding and scripting of learning, as it is coined by here, at LMU, appears more and more as a must and not an option. Second, in a more naïve and perhaps a bit emphatic approach, there is no field that comes close in terms of complexity than Learning Sciences. The ‘latent’ or ‘implicit’ nature of its constructs, as well as its rich implications for science and society, make Learning Sciences the ‘ideal’ ground for using the tremendous power of Machine Learning and Artificial Intelligence. If there is one conclusion that I allow myself, is dare saying that Learning Analytics is not a possible and very promising future, but a very stringent present.
References
Fischer, F., Hmelo-Silver, C. E., Goldman, S. R., & Reimann, P. (2018). International handbook of the learning sciences. Routledge New York, NY.
Goldstein, P. J., & Katz, R. N. (2005). Academic analytics: The uses of management information and technology in higher education (Vol. 8). Educause.
Siemens, G., & Baker, R. S. d. (2012). Learning analytics and educational data mining: Towards communication and collaboration. In Proceedings of the 2nd international conference on learning analytics and knowledge (pp. 252–254).
Last modified on 2021-04-07