lastmod: 11 May, 2020
A brief disambiguation
One of the most important sources of confusion, especially for those coming from a social sciences background, which are usually trained (first) in Statistics, is where does this ‘machine learning’ (ML) comes from and what is it’s relation with the ‘classical’ statistics they are accustomed with.
The following is, undoubtedly, a very limitted and superficial approach, and there are many aspects to consider when attempting a proper analysis and comparison of the two, but it’s a start.
How do they compare
Science has two main roles. The first is to explain why things happen in a certain way. Second, to make predictions, to indicate what will happen in the future given the data from the present.
For instance, given what we know about these (known) variables, what is likely to happen to that (unknown) outcome/response variable. Similarly important is the second question, why do things happens in this (observed) way.
Hence, in the sense that scientific theories are validated by their predictive power, in my personal opinion, both are proper tools for doing science.
However, machine learning algorithm are better equiped to answer the ‘what’ question, whereas (classical) statistics is more suited for the ‘why’.
For instance, what patterns, what variables/features, what outcome, etc. versus why do two aspects/traits covary, why this outcome, etc.
Machine learning is less interested in establishing generalizability to population and more concerned with the practical significannce of the result, i.e., with the accuracy of the prediction. This can be seen by noticing that its approach is less constrained by assumptions regarding the distributions and the parameters.
This doesn’t mean that the result of a machine learning algorithm cannot hold true for the population. It only means that it does so if the sample that provided the data used by said algorithm is representative.
Where do they come from
Statistics (as everyone knows, right?) is a subfield of mathematics. Its roots are centuries old and so are some of its main techniques. It is (still) the backbone of social science research, albeit its predominance is beginning to be challenged by the tremendous development of machine learning and other computational approaches.
Machine learning, on the other hand, is a relatively new field of practice. It is a subfield of computer science and its huge raise in popularity and use is mostly due to the technological developments of the last few decades as well as to the increased access of regular users to said new computational technologies.
ML vs Statistics in pictures
They say a picture is worth a thousand words, and I couldn’t agree more. So, here are a few thousand words in the form of pictures, that can help understand the relation between machine learning and statistics.
Note: click on the linked lables of the pictures below to read more about several visual representations of classifications of machine learning algorithms on various web sites that explore this subject in more depth.
And a personal note
In addition to the above, in my own understanding, I feel that ML is also a very useful tool for exploring possible patterns. This doesn’t diminish in the least its value as a predictive tool. Rather, I feel it adds to its applicability and to its power to complement the ‘traditional’ approaches.
The ‘traditional’ depiction
Note: the example below comes from the ‘data science’ approach, whose proponents tend to view it as the overlap of three main fields.
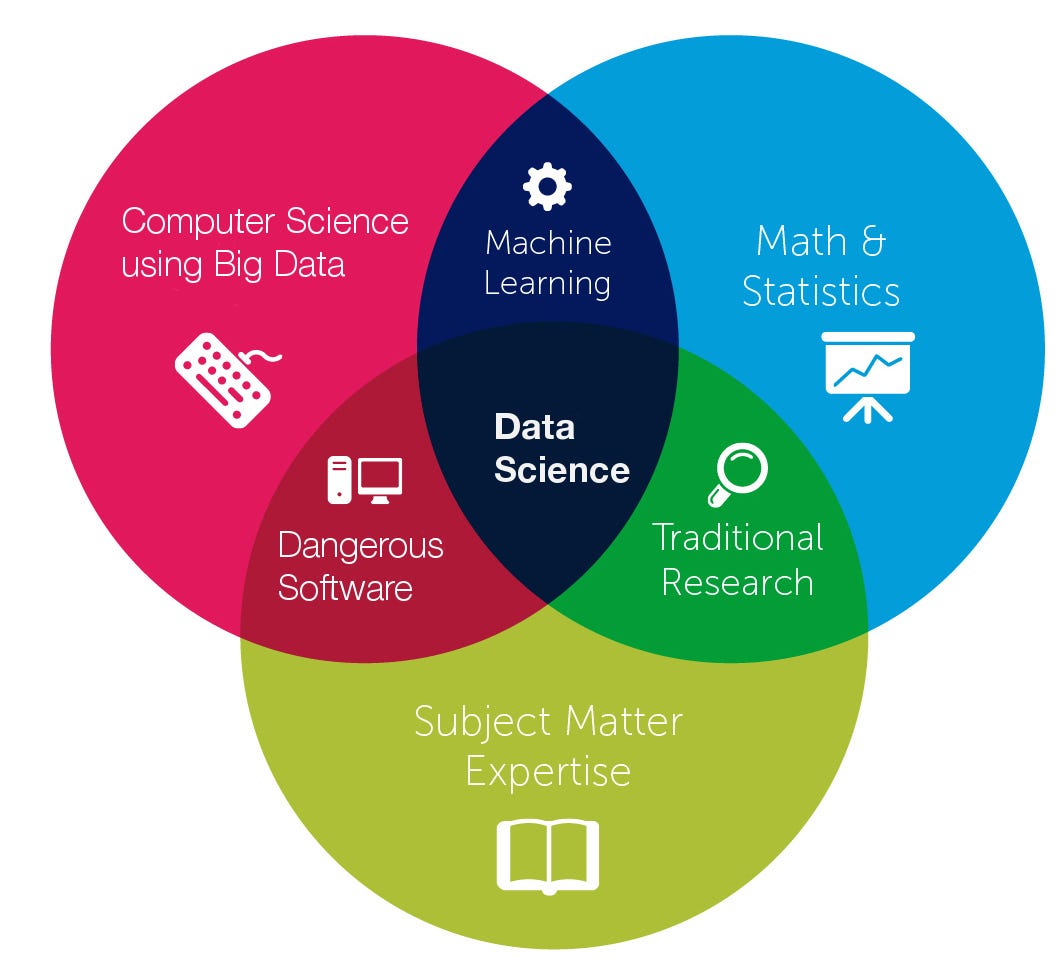
source: Medium
Vs. a couple of more ‘affirmative’ examples
Note: the two examples below are specific to the ‘data mining’ community, who tends to view data mining as the overlap of several fields, including Statistics and ML

source: AI Theology
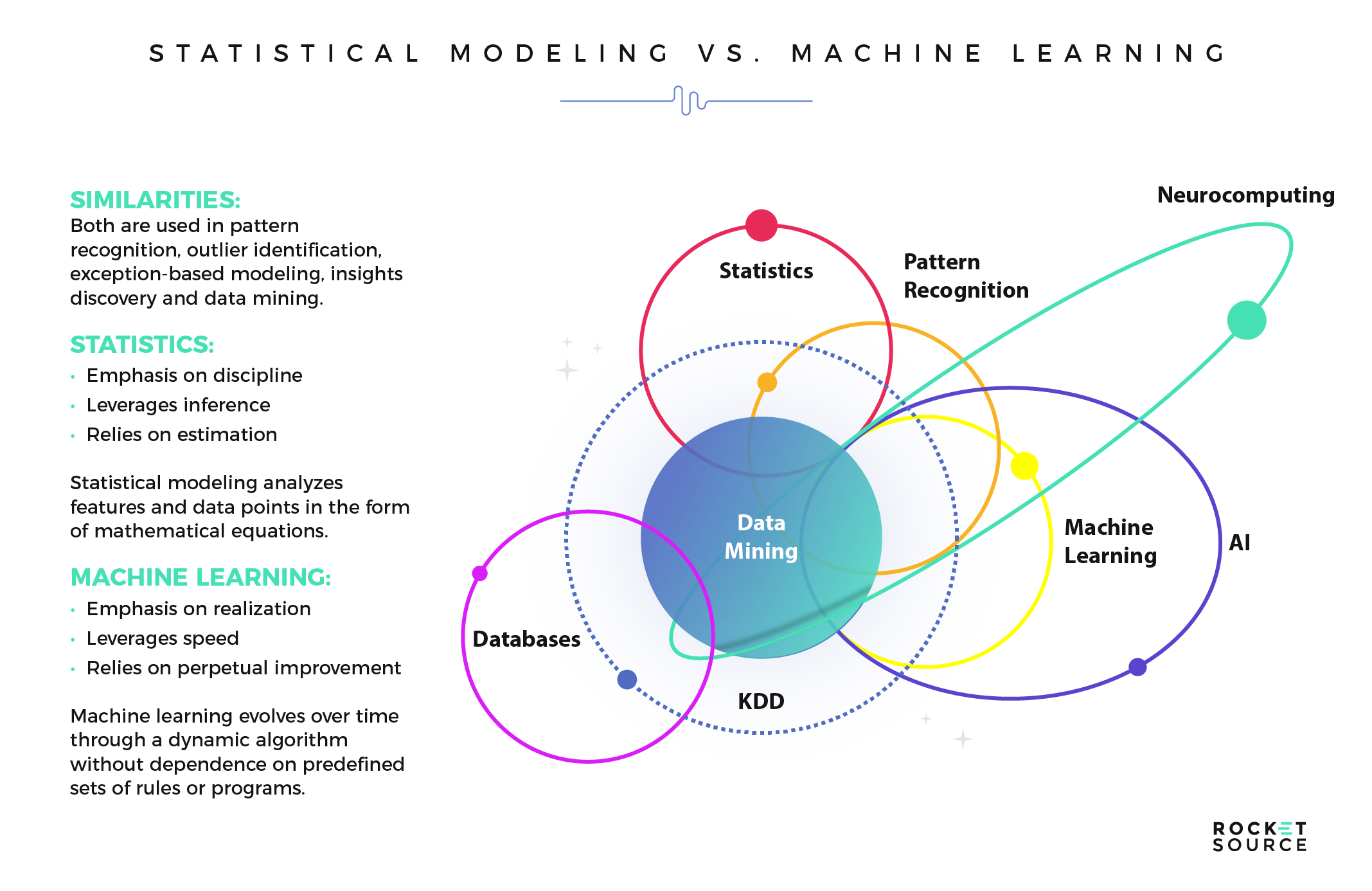
source: Rocket Source
Last modified on 2021-04-07